
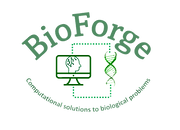
Gene expression analysis
Role of AI in gene expression analysis
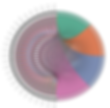
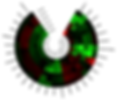

Gene expression analysis is a multifaceted field that plays a crucial role in understanding how genes are transcribed and translated into functional proteins. This process involves a combination of wet lab experiments, bioinformatics, and Artificial Intelligence (AI) techniques to gain a comprehensive insight into the dynamic and complex regulatory mechanisms governing gene expression.
In the wet lab, researchers perform various experimental techniques to quantify and characterize gene expression levels. One of the fundamental methods is RNA sequencing (RNA-seq), which allows scientists to identify and quantify the transcriptome of a cell or tissue. This technology provides a global view of gene expression and helps identify differentially expressed genes under various conditions, such as disease states or drug treatments. Additionally, techniques like microarrays and qPCR are also used to measure gene expression levels, providing complementary data to RNA-seq.
Following data generation, bioinformatics takes center stage to process, analyze, and interpret the vast amount of gene expression data. This involves preprocessing steps to remove noise and artifacts, aligning and mapping sequencing reads to a reference genome, and quantifying expression levels. Differential gene expression analysis identifies genes with altered expression between experimental groups, shedding light on potential key regulators and biological pathways. Network analysis and functional enrichment methods allow researchers to understand the underlying biological processes and interactions associated with differentially expressed gene
The integration of Artificial Intelligence, particularly machine learning algorithms, significantly enhances gene expression analysis. AI-based models can accurately predict gene expression patterns and identify biomarkers associated with specific diseases or conditions.
Deep learning techniques, such as convolutional neural networks (CNNs) and recurrent neural networks (RNNs), excel in deciphering complex gene expression patterns and identifying novel gene regulatory elements. Furthermore, AI helps in clustering and classifying samples based on gene expression profiles, enabling the discovery of distinct subtypes or phenotypes within a disease.
Moreover, AI-driven approaches enable the prediction of transcription factor binding sites, promoter regions, and other regulatory elements, facilitating a deeper understanding of gene regulatory networks. This knowledge is crucial for unraveling the intricate interplay between genes and their regulatory mechanisms. AI algorithms can also integrate multi-omics data, such as DNA methylation and chromatin accessibility, to provide a more comprehensive picture of gene regulation.
Here at BioForge, we are providing gene expression analysis with wet lab experiments, bioinformatics, and AI. These approaches together enable researchers to decode the intricate language of gene expression, revealing key insights into various biological processes, disease mechanisms, and potential therapeutic targets. As technology and methodologies continue to advance, gene expression analysis will undoubtedly remain at the forefront of molecular biology and precision medicine research.